Why in the News?
- Earlier this year, a South Korean lab announced a breakthrough that could be “a possible real solution to the energy crisis”.
What’s in Today’s Article?
- Background (Context)
- About Google DeepMind’s Project (Objective, Working Mechanism, Significance)
Background:
- Scientists at the Seoul-based Korea University in July presented lumps of a grey-black, polycrystalline compound known as LK-99.
- The scientists claimed that the LK-99 could be fused to fabricate a superconductor that worked at room temperature and at normal pressure.
- However, these claims subsequently didn’t survive the test of scrutiny.
- Inspired by the research, Google announced a new project on the similar lines.
About Google DeepMind’s Project:
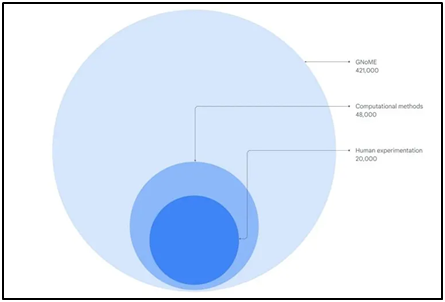
- Google announced the launch of DeepMind AI tool, known as Graph Networks for Materials Exploration (GNoME).
- It used Artificial Intelligence (AI) to predict the structures of more than 2 million new materials.
- This could have wide-reaching application in sectors such as renewable energy, battery research, semiconductor design and computing efficiency.
How does GNoME Work?
- GNoME is a state-of-the-art graph neural network model or GNN, where the input data for the model takes the form of a graph that can then be likened to connections between atoms.
- GNoME was trained using “active learning”, a technique to scale up a model first trained on a small specialised dataset.
- Developers can then introduce new targets allowing machine learning to label new data with human assistance.
- This makes the algorithm “well suited” to the science of discovering new materials, which requires searching for patterns not found in the original dataset.
- Working Mechanism of GNoME:
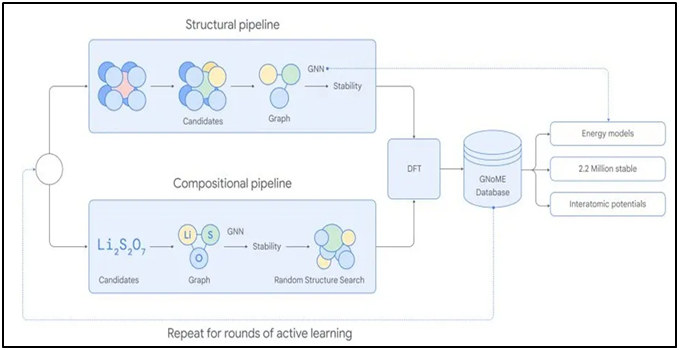
- As seen here, GNoME uses two pipelines to discover low-energy (stable) materials.
- The structural pipeline creates candidates with structures similar to known crystals, while the compositional pipeline follows a more randomized approach based on chemical formulas.
- The outputs of both pipelines are evaluated using established Density Functional Theory calculations and those results are added to the GNoME database, informing the next round of active learning.
- As a result, the model has boosted the precision rate for predicting materials stability from 50 per cent to around 80 per cent.
- DeepMind claims its current research is equivalent to nearly 800 years of knowledge, given that 380,000 of its stable predictions are now publicly available to help researchers make further breakthroughs in materials discovery teams.
Significance of GNoME:
- In one shot, this AI-linked breakthrough increases the number of ‘stable materials’ known to mankind ten-fold.
- These materials include inorganic crystals that modern tech applications from computer chips to batteries rely on.
- To enable new technologies, crystals must be stable — otherwise they can simply decompose.
- While these materials will still need to undergo the process of synthesis and testing, DeepMind has published a list of 381,000 of the 2.2 million crystal structures that it predicts to be most stable.
- Over the last decades, experimentation by humans has resulted in the discovery of the structures of some 28,000 stable materials, which are listed in the Inorganic Crystal Structures Database, the largest database of identified materials.